Chapter 0. Randomness
“The generation of random numbers is too important to be left to chance.”
— Robert R. Coveyou
Here we are: the beginning. If it’s been a while since you’ve programmed in JavaScript (or done any math, for that matter), this chapter will reacquaint your mind with computational thinking. To start your coding of nature journey, I’ll introduce you to some foundational tools for programming simulations: random numbers, random distributions, and noise. Think of this as the first (zeroth!) element of the array that makes up this book—a refresher and a gateway to the possibilities that lie ahead.
In Chapter 1, I’m going to talk about the concept of a vector and how it will serve as the building block for simulating motion throughout this book. But before I take that step, let’s think about what it means for something to move around a digital canvas. I’ll begin with one of the best-known and simplest simulations of motion: the random walk.
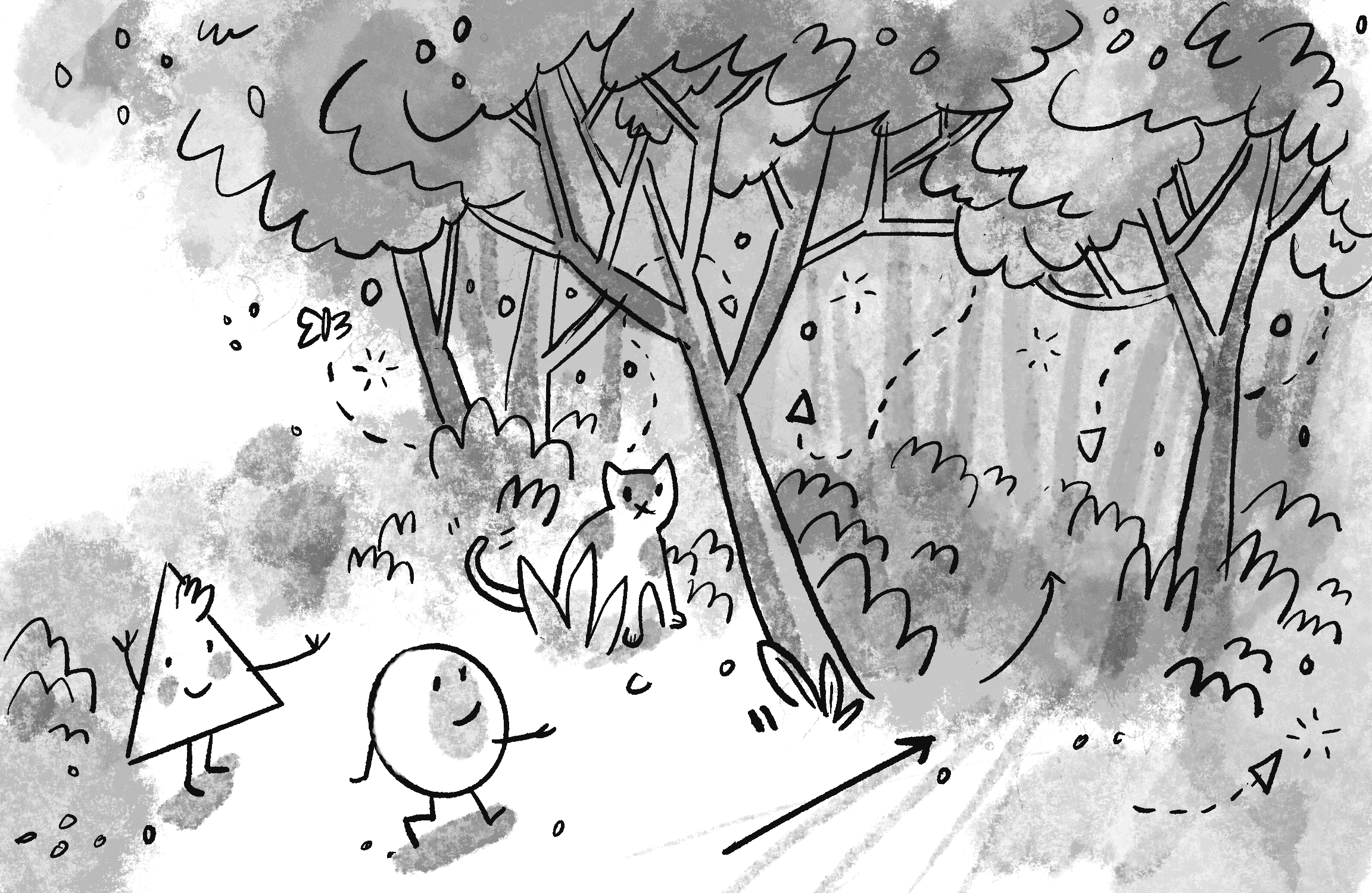
Random Walks
Imagine you’re standing in the middle of a balance beam. Every ten seconds, you flip a coin. Heads, take a step forward. Tails, take a step backward. This is a random walk, a path defined as a series of random steps. Stepping (carefully) off that balance beam and onto the floor, you could perform a random walk in two dimensions by flipping the same coin twice with the following results:
Flip 1 | Flip 2 | Result |
---|---|---|
Heads | Heads | Step forward. |
Heads | Tails | Step right. |
Tails | Heads | Step left. |
Tails | Tails | Step backward. |
This may seem like an unsophisticated algorithm, but you can use random walks to model all sorts of phenomena that occur in the real world, from the movements of molecules in a gas, to an animal foraging for food, to the behavior of a gambler spending a day at the casino. For our purposes, the random walk is the perfect place to start for three reasons:
- I’d like to review a programming concept central to this book: object-oriented programming. The random walker I’m about to create will serve as a template for how I’ll use object-oriented design to make things that move around a computer graphics canvas.
- The random walk instigates the two questions that I’ll ask over and over again throughout this book: “How do you define the rules that govern the behavior of your objects?” and then, “How do you implement these rules in code?”
- You’ll periodically need a basic understanding of randomness, probability, and Perlin noise for this book’s projects. The random walk will allow me to demonstrate key points that will come in handy later.
I’ll first review a bit of object-oriented programming (OOP) by coding a Walker
class to create Walker
objects that can go for a random walk. This will only be a cursory review. If you’ve never worked with OOP before, you may want something more comprehensive; I’d suggest stopping here and reviewing this video tutorial on the basics of ES6 classes with p5.js before continuing.
The Random Walker Class
An object in JavaScript is an entity that has both data and functionality. In this case, a Walker
object should have data about its position on the canvas and functionality such as the capability to draw itself or take a step.
A class is the template for building actual instances of objects. Think of a class as the cookie cutter and objects as the cookies themselves. To create a Walker
object, I’ll begin by defining the Walker
class—what it means to be a Walker
.
The Walker
only needs two pieces of data: a number for its x-position and one for its y-position. I’ll initialized them to the center of the canvas to set the object’s starting position. I can do this in the class’s constructor function, appropriately named constructor()
. You can think of the constructor as the object’s setup()
function. It’s responsible for defining the initial properties of an object, much like setup()
does for the entire sketch.
constructor() {
Objects have a constructor where they are initialized.
this.x = width / 2;
this.y = height / 2;
}
Objects have data.
Notice the use of the keyword this
to attach the properties to the newly created object itself: this.x
and this.y
.
In addition to data, classes can be defined with functionality. In this example, a Walker
object has two functions, known as methods in an OOP context. While methods are essentially functions, the distinction is that methods are defined inside of a class, and so are associated with an object or class, whereas functions aren’t. The function
keyword is a nice clue: you'll see it when defining standalone functions, but it won’t appear inside a class. I’ll try my best to use the terms consistently in this book, but it’s common for programmers to use the terms “function” and “method” interchangeably.
The first method, show()
, includes the code to draw the object (as a black dot). Once again, never forget the this.
when referencing the properties (variables) of that object.
show() {
stroke(0);
point(this.x, this.y);
}
Objects have methods.
The next method, step()
, directs the Walker
object to take a step. This is where things get a bit more interesting. Remember taking steps in random directions on a floor? Now I’ll use a p5.js canvas to represent that floor. There are four possible steps. A step to the right can be simulated by incrementing x
with x++
; to the left by decrementing x
with x--
; forward by going up a pixel (y--
); and backward by going down a pixel (y++
). But how can the code pick from these four choices?
Earlier I stated that you could flip two coins. In p5.js, however, when you want to randomly choose from a list of options, you can simply generate a random number with the random()
function. It picks a random floating point (decimal) value within any range you want.
let choice = floor(random(4));
Here I declare a variable choice
and assign it a random integer (whole number) with a value of 0, 1, 2, or 3 by removing the decimal places from the random floating point number using floor()
. Technically speaking, the number picked by calling random(4)
can never be 4.0, since the top end of the range isn’t inclusive. Rather, the highest possibility is 3.999999999 (with as many 9s as JavaScript will allow), which floor()
will round down to 3.
Declaring Variables
In JavaScript, variables can be declared using either let
or const
. A typical approach would be to declare all variables with const
and change to let
when needed. In this first example, const
would be appropriate for declaring choice
as it’s never reassigned a new value over the course of its life inside each call to step()
. While this differentiation is important, I’m choosing to follow the p5.js example convention and declare all variables with let
. I recognize there are important reasons for having const
and let
. However, the distinction can be a distraction and confusing for beginners. I encourage you, the reader, to explore the topic further and make your own decisions about how to best declare variables in your own sketches. For more, you can read the discussion surrounding issue #3877 in the p5.js GitHub repository.
While I'm at it, I'm also choosing to use the "strict" equality boolean operator in JavaScript: ===
. This operator tests both value and type equality. For example, 3 === '3'
will evaluate to false
because the types are different (number vs. string), even though they look similar. On the other hand, using ==
in 3 == '3'
will result in true
because the two different types are converted to be comparable, which can sometimes lead to unexpected results. Although the loose comparison ==
would work fine here, ===
is probably a safer option.
Next, the walker takes the appropriate step (left, right, up, or down), depending on which random number was picked. Here’s the full step()
method closing out the Walker
class.
step() {
let choice = floor(random(4));
0, 1, 2, or 3
if (choice === 0) {
this.x++;
} else if (choice === 1) {
this.x--;
} else if (choice === 2) {
this.y++;
} else {
this.y--;
}
}
}
The random "choice" determines the step.
Now that I’ve written the class, it’s time to make an actual Walker
object in the sketch itself. Assuming you’re looking to model a single random walk, start with a single global variable.
let walker;
A Walker object
Then create the object in setup()
by referencing the class name with the new
operator.
function setup() {
Remember how p5.js works? setup() is executed once when the sketch starts...
createCanvas(640, 240);
walker = new Walker();
background(255);
}
Create the Walker.
Finally, during each cycle through draw()
, the Walker
takes a step and draws a dot.
function draw() {
...and draw() loops forever and ever (until you quit).
walker.step();
walker.show();
}
Call functions on the Walker.
Since the background is drawn once in setup()
, rather than clearing it continually each time through draw()
, the trail of the random walk is visible in the canvas.
Example 0.1: A Traditional Random Walk
There are a couple adjustments I could make to the random walker. For one, this Walker
object’s steps are limited to four options: up, down, left, and right. But any given pixel in the canvas can be considered to have eight possible neighbors, including diagonals (see Figure 0.1). A ninth possibility to stay in the same place could also be an option.
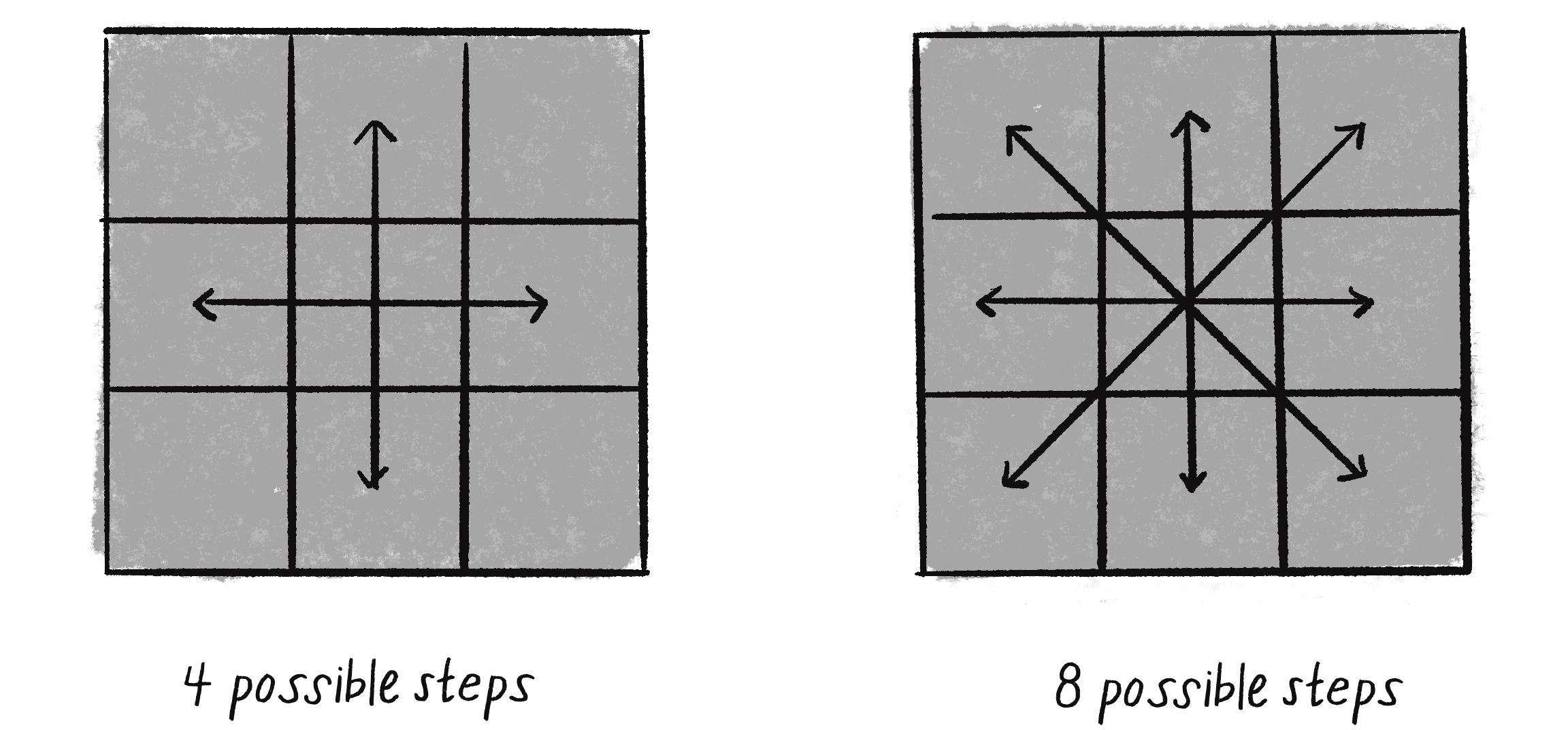
To implement a Walker
object that can step to any neighboring pixel (or stay put), I could pick a number between 0 and 8 (nine possible choices). However, another way to write the code would be to pick from three possible steps along the x-axis (-1, 0, or 1) and three possible steps along the y-axis.
step() {
let xstep = floor(random(3)) - 1;
let ystep = floor(random(3)) - 1;
Yields -1, 0, or 1
this.x += xstep;
this.y += ystep;
}
Taking this further, I could get rid of floor()
and use the random()
function’s original floating point numbers to create a continuous range of possible step lengths between -1 and 1.
step() {
let xstep = random(-1, 1);
let ystep = random(-1, 1);
Any floating point number between -1.0 and 1.0
this.x += xstep;
this.y += ystep;
}
All of these variations on the “traditional” random walk have one thing in common: at any moment in time, the probability that the Walker
will take a step in a given direction is equal to the probability that the Walker
will take a step in any other direction. In other words, if there are four possible steps, there is a 1 in 4 (or 25 percent) chance the Walker
will take any given step. With nine possible steps, it’s a 1 in 9 chance (about 11.1 percent).
Conveniently, this is how the random()
function works. p5’s random number generator (which operates behind the scenes) produces a uniform distribution of numbers. You can test this distribution with a sketch that counts each time a random number is picked and graphs it as the height of a rectangle.
Example 0.2: A Random Number Distribution
let randomCounts = [];
An array to keep track of how often random numbers are picked
let total = 20;
function setup() {
createCanvas(640, 240);
for (let i = 0; i < total; i++) {
randomCounts[i] = 0;
}
}
function draw() {
background(255);
Total number of slots
let index = floor(random(randomCounts.length));
randomCounts[index]++;
stroke(0);
fill(127);
let w = width / randomCounts.length;
Pick a random number and increase the count.
for (let x = 0; x < randomCounts.length; x++) {
rect(x * w, height - randomCounts[x], w - 1, randomCounts[x]);
}
}
Graphing the results
Notice how each bar of the graph differs slightly in height. The sample size (the number of random numbers picked) is small, so occasional discrepancies where certain numbers are picked more often emerge. Over time, with a good random number generator, this would even out.
Pseudorandom Numbers
The random numbers from the random()
function aren’t truly random; instead, they’re known as pseudorandom because they’re the result of a mathematical function that merely simulates randomness. This function would yield a pattern over time, and thus stop seeming to be random. That time period is so long, however, that random()
is random enough for the examples in this book.
Exercise 0.1
Create a random walker that has a greater tendency to move down and to the right. (The solution follows in the next section.)
Probability and Nonuniform Distributions
Uniform randomness often isn’t the most thoughtful solution to a design problem—in particular, the kind of problem that involves building an organic or natural-looking simulation. With a few tricks, however, the random()
function can instead produce nonuniform distributions of random numbers, where some outcomes are more likely than others. This can yield more interesting, seemingly natural results.
Think about when you first started programming with p5.js. Perhaps you wanted to draw a lot of circles on the screen, so you said to yourself, “Oh, I know! I’ll draw all these circles at random positions, with random sizes and random colors.” Seeding a system with randomness is a perfectly reasonable starting point when you’re learning the basics of computer graphics, but in this book, I’m looking to build systems modeled on what we see in nature, and uniform randomness won’t always cut it. Sometimes you have to put your thumb on the scales a little bit.
Creating a nonuniform distribution of random numbers will come in handy throughout the book. In Chapter 9’s genetic algorithms, for example, I’ll need a methodology for performing “selection”—which members of the population should be selected to pass their DNA to the next generation? This is akin to the Darwinian concept of “survival of the fittest.” Say you have an evolving population of monkeys. Not every monkey has an equal chance of reproducing. To simulate Darwinian natural selection, you can’t simply pick two random monkeys to be parents. The more “fit” ones should be more likely to be chosen. This could be considered the “probability of the fittest.”
Let me pause here and take a look at probability’s basic principles, so I can apply more precise words to the coding examples to come. I’ll start with single-event probability—the likelihood that a given event will occur. In probability, outcomes refer to all the possible results of a random process, and an event is the specific outcome or combination of outcomes being considered.
If you have a scenario where each outcome is just as likely as the others, the probability of given event occurring equals the number of outcomes that match that event divided by the total number of all potential outcomes. A coin toss is a simple example: it has only two possible outcomes, heads or tails. There’s only one way to flip heads, so the probability that the coin will turn up heads is one divided by two: 1/2, or 50 percent.
Take a deck of 52 cards. The probability of drawing an ace from that deck is:
The probability of drawing a diamond is:
You can also calculate the probability of multiple events occurring in sequence by multiplying the individual probabilities of each event. For example, the probability of a coin turning up heads three times in a row is:
This indicates a coin will turn up heads three times in a row one out of eight times on average. If you flipped a coin three times in a row 500 times, you would expect to see an outcome of three consecutive heads an average of one-eighth of the time, or about 63 times.
Exercise 0.2
What is the probability of drawing two aces in a row from a deck of 52 cards, if you reshuffle your first draw back into the deck before making your second draw? What would that probability be if you didn’t reshuffle after your first draw?
There are a couple of ways to use the random()
function to apply the concepts of probability in your code for a nonuniform distribution. One technique is to fill an array with numbers—some of which are repeated—then choose random elements from that array and generate events based on those choices.
let stuff = [1, 1, 2, 3, 3];
1 and 3 are stored in the array twice, making them more likely to be picked than 2.
let value = random(stuff);
Picking a random element from an array
print(value);
The five-member array has two 1s, so running this code will produce a 2/5 = 40 percent chance of printing the value 1. Likewise, there’s a 20 percent chance of printing 2, and a 40 percent chance of printing 3.
You can also ask for a random number (let’s make it simple and just consider random floating point values between 0 and 1) and allow an event to occur only if the random number is within a certain range. For example:
let probability = 0.1;
A probability of 10%
let r = random(1);
A random floating point between 0 and 1
if (r < probability) {
print('Sing!');
}
If the random number is less than 0.1, sing!
One-tenth of the floating point numbers between 0 and 1 are less than 0.1, so this code will only lead to singing 10 percent of the time.
You can use the same method to apply unequal weights to multiple outcomes. Let’s say you want singing to have a 60 percent chance of happening, dancing, a 10 percent chance, and sleeping, a 30 percent chance. Again, you can pick a random number between 0 and 1 and see where it falls:
- Between 0.0 and 0.6 (60 percent) → Singing
- Between 0.6 and 0.7 (30 percent) → Dancing
- Between 0.7 and 1.0 (30 percent) → Sleeping
let num = random(1);
if (num < 0.6) {
print("Sing!");
If random number is less than 0.6
} else if (num < 0.7) {
print("Dance!");
Between 0.6 and 0.7
} else {
print("Sleep!");
}
Greater than 0.7
Now let’s apply this methodology to the random walker so it tends to move in a particular direction. Here’s an example of a Walker
with the following probabilities:
- chance of moving up: 20 percent
- chance of moving down: 20 percent
- chance of moving left: 20 percent
- chance of moving right: 40 percent
Example 0.3: A Walker That Tends to Move to the Right
step() {
let r = random(1);
if (r < 0.4) {
this.x++;
A 40% chance of moving to the right!
} else if (r < 0.6) {
this.x--;
} else if (r < 0.8) {
this.y++;
} else {
this.y--;
}
}
Another common use of this technique is to control the probability of an event that you want to occur sporadically in your code. For example, let’s say you create a sketch that starts a new random walker at regular time intervals (every 100 frames). With random()
you could instead assign a 1 percent chance of a new walker starting. The end result is the same (a new walker every 1 out of 100 frames on average), but the latter incorporates chance and feels more dynamic and unpredictable.
Exercise 0.3
Create a random walker with dynamic probabilities. For example, can you give it a 50 percent chance of moving in the direction of the mouse? Remember, you can use mouseX
and mouseY
to get the current mouse position in p5.js!
A Normal Distribution of Random Numbers
Another way to create a nonuniform distribution of random numbers is to use a normal distribution, where the numbers cluster around an average value. To see why this is useful, let’s go back to that population of simulated monkeys and assume your sketch generates a thousand Monkey
objects, each with a random height value between 200 and 300 (as this is a world of monkeys that have heights between 200 and 300 pixels).
let h = random(200, 300);
Is this an accurate algorithm for creating a population of monkey heights? Think of a crowded sidewalk in New York City. Pick any person off the street and it may appear that their height is random. Nevertheless, it’s not the kind of random that random()
produces by default. People’s heights aren’t uniformly distributed; there are many more people of about average height than there are very tall or very short ones. To accurately reflect this, random heights close to the mean (another word for “average”) should be more likely to be chosen, while outlying heights (very short or very tall) should be more rare.
That’s exactly how a normal distribution (sometimes called a “Gaussian distribution” after mathematician Carl Friedrich Gauss) works. A graph of this distribution, informally known as a “bell” curve, is shown in Figure 0.2.
The curve is generated by a mathematical function that defines the probability of any given value occurring as a function of the mean (often written as μ, the Greek letter mu) and standard deviation (σ, the Greek letter sigma).
In the case of height values between 200 and 300, you probably have an intuitive sense of the mean (average) as 250. However, what if I were to say that the standard deviation is 3? Or 15? What does this mean for the numbers? The graph depicted in Figure 0.2 should give you a hint. The standard deviation changes over time. When the animation begins, it shows a high peak. This is a distribution with a very low standard deviation, where the majority of the values pile up around the mean (they don’t deviate much from the standard). As the standard deviation increases, the values spread out more evenly from the average (since they’re more likely to deviate).
The numbers work out as follows: Given a population, 68 percent of the members of that population will have values in the range of one standard deviation from the mean, 95 percent within two standard deviations, and 99.7 percent within three standard deviations. Given a standard deviation of 5 pixels, only 0.3 percent of the monkey heights will be less than 235 pixels (three standard deviations below the mean of 250) or greater than 265 pixels (three standard deviations above the mean of 250). Meanwhile, 68 percent of monkey heights will be between 245 and 255 pixels.
Calculating Mean and Standard Deviation
Consider a class of ten students who receive the following scores (out of 100) on a test:
The mean is the average:
The standard deviation is calculated as the square root of the average of the squares of deviations around the mean. In other words, take the difference between the mean and each person’s grade, and square it, giving you that person's “squared deviation.” Next, calculate the average of all these values to get the average variance. Then, take the square root of the average variance, and you have the standard deviation.
Score | Difference from Mean | Variance |
---|---|---|
class Walker {